May 10, 20229 min read
132


Dec 24, 20214 min read
85
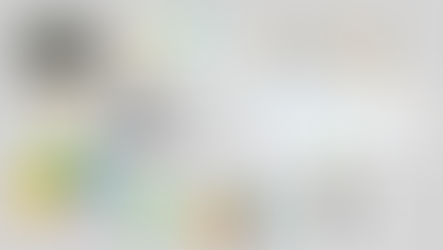

Oct 27, 20183 min read
54
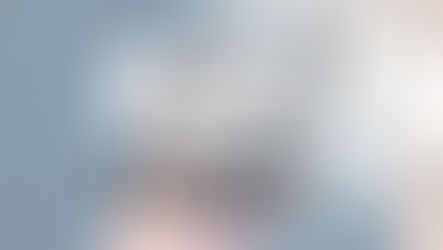

Updated: Apr 30, 2023
.
You may visualise the fight against financial crime as one managed in two main parts in the lifecycle of a customer. First when the customer is on-boarded, and a KYC is carried out to understand who the customer is. The other is after on-boarding, the ongoing monitoring of the customer to ensure the activities of the customer are in line with acceptable norms. This ongoing monitoring can again be divided into two main parts - monitoring the transactions of the customer and the other gaining better insights about the customer from information like emerging new relationships, business associations, and many other data points from diverse sources including social media. When these are analysed accurately they speak a lot about who the customers are and how do they carry out their business activities?
Now traditionally, banks are engaged in transaction-based monitoring for detection of any criminal activities on an ongoing basis. The limitation of this process is that it creates a lot of false positives and resources and efforts are wasted in managing them. The overall effectiveness of the process is nowhere comparable to the magnitude of the problem of financial crime. Only 1% of financial crime through the banking system is stopped, and it creates waste for banks, destroys trust and impacts society globally.
Technology has given us a tool and a hope to fight against this enormous challenge we have today. We see intelligence-led approach for ongoing monitoring of customer transactions enabled by big data, artificial intelligence and machine learning as an effective solution to study patterns and identify hidden anomalies. We already have piles of historical transaction data which if used effectively to train the machines and create models we can use these models or algorithms to test new sets of transaction data on an ongoing basis. The benefits of using machines to test transaction data are phenomenal. We can achieve a 100% test coverage for all new transactions at a very rapid pace and in a cost-effective manner with high levels of accuracy.
The other part of the solution would be using machines to gain deeper and better insights about the customers. This would be possible by machines producing artificial neural networks linking millions of data points from seemingly unrelated databases containing everything from social media posts to internet protocol addresses used on airport Wi-Fi networks to real estate holdings or tax returns, relationships and business associations, etc. to identify patterns. This knowledge would be very beneficial to draw risk profile of the entities enabling banks to focus on that small section of their customers who have the risk profile beyond a threshold level. This will enable the other large majority of the customers who need not be bothered for going through the cumbersome vigilance process and they can enjoy an easy & smooth experience.
Again the benefits are beyond imagination wherein large volumes of diverse information will be used to analyse customer behaviour. This knowledge would be truly beneficial to set the level of vigilance needed for different groups of customers and take appropriate actions based on the alerts raised by the transaction analytics part of the solution. Timely action by sharing the information with adequate details with the right people internally and externally would be effective in containing the crime.
There are indeed challenges in implementing this solution at the same time many favourable factors. As we see the models and the neural networks are the creation of the data, they are fed with. Hence the quality of the data is fundamental to this solution. The favourable part is that there is a vast amount of data for transactions anomaly detection already available. With our application "Compliance by Design" that will be used across the bank and it's geographies availability of this data will be further simplified. These large volumes of data need very high computing power for their analysis. Availability of such levels of computing power is now a reality already. The challenge would be in developing the models that would be fit for the purpose. Now collecting adequate data for business’s ongoing behaviour analysis will be challenging as it is constantly changing and a detailed and comprehensive view of that would look like what is not known yet. Possibilities of bias in that data and lack of explainability of the models if not addressed adequately would undermine the credibility of the predictions.
Given all these challenges and constraints Machine Learning is still a glittering hope in solving this big challenge facing the financial system today.
Comments